Prevención y gestión de accidentes en la minería subterránea peruana: aplicación de inteligencia artificial y machine learning
Palabras clave:
Prevención y gestión, accidentes, minería subterráneaSinopsis
La minería ha sido desde hace mucho tiempo un motor crucial para el desarrollo económico de muchos países, en especial de Perú, generando empleo, recursos y crecimiento económico en distintas regiones. No obstante, el progreso viene acompañado de riesgos, y la minería subterránea destaca como una de las actividades industriales más peligrosas debido a las condiciones extremas y los desafíos de seguridad inherentes a sus operaciones. Este libro nace de la convicción de que, a través de la investigación científica y la innovación tecnológica, es posible transformar la gestión de seguridad en este sector, protegiendo tanto a los trabajadores como a las comunidades circundantes.
A lo largo de estas páginas, se aborda una visión integral sobre la prevención de accidentes laborales en la minería subterránea peruana, utilizando herramientas avanzadas como la inteligencia artificial y el machine learning. La investigación y análisis presentados representan años de trabajo dedicado a comprender los factores críticos de riesgo en esta industria y a diseñar soluciones que ayuden a anticipar y reducir la ocurrencia de accidentes. Este libro está destinado a todos aquellos interesados en mejorar la seguridad laboral y la gestión de riesgos, incluidos investigadores, profesionales de la minería, reguladores, y estudiantes de ingeniería y tecnología. Con el respaldo de datos y metodologías innovadoras, esperamos que esta obra contribuya a un cambio positivo y duradero en la industria minera peruana.
Descargas
Referencias
Abdalzaher, M. S., Elsayed, H. A., Fouda, M. M., & Salim, M. M. (2023). Employing Machine Learning and IoT for Earthquake Early Warning System in Smart Cities. Energies, 16(1), 1–22. https://doi.org/10.3390/en16010495
Abdul Aziz, H., & Mohd Shariff, A. (2017). A Journey of Process Safety Management Program for Process Industry. International Journal of Engineering Technology and Sciences, 4(2), 119–127. https://doi.org/10.15282/ijets.8.2017.1.10.1085
Adaku, E., Ankrah, N. A., & Ndekugri, I. E. (2021). Design for occupational safety and health : A theoretical framework for organisational capability. Safety Science, 133(September 2020), 105005. https://doi.org/10.1016/j.ssci.2020.105005
Ahadh, A., Binish, G. V., & Srinivasan, R. (2021). Text mining of accident reports using semi-supervised keyword extraction and topic modeling. Process Safety and Environmental Protection, 155, 455–465. https://doi.org/10.1016/j.psep.2021.09.022
Ahmad, S. B. S., Mazhar, M. U., Bruland, A., Andersen, B. S., Langlo, J. A., & Torp, O. (2020). Labour productivity statistics: a reality check for the Norwegian construction industry. International Journal of Construction Management, 20(1), 39–52. https://doi.org/10.1080/15623599.2018.1462443
Akanmu, A. A., Olayiwola, J., Ogunseiju, O., & McFeeters, D. (2020). Cyber-physical postural training system for construction workers. Automation in Construction, 117(May), 103272. https://doi.org/10.1016/j.autcon.2020.103272
Alawamleh, M., & Popplewell, K. (2011). Interpretive structural modelling of risk sources in a virtual organisation. International Journal of Production Research, 49(20), 6041–6063. https://doi.org/10.1080/00207543.2010.519735
Ali, N. F. M., Sadullah, A. F. M., Majeed, A. P. P. A., Razman, M. A. M., & Musa, R. M. (2022). The identification of significant features towards travel mode choice and its prediction via optimised random forest classifier: An evaluation for active commuting behavior. Journal of Transport & Health, 25, 101362.
Almeida, C. S. de, Miccoli, L. S., Andhini, N. F., Aranha, S., Oliveira, L. C. de, Artigo, C. E., Em, A. A. R., Em, A. A. R., Bachman, L., Chick, K., Curtis, D., Peirce, B. N., Askey, D., Rubin, J., Egnatoff, D. W. J., Uhl Chamot, A., El‐Dinary, P. B., Scott, J.; Marshall, G., Prensky, M., … Santa, U. F. De. (2016a). Budgeting for Local Governments and Communities. In Revista Brasileira de Linguística Aplicada (Vol. 5, Issue 1).
Almeida, C. S. de, Miccoli, L. S., Andhini, N. F., Aranha, S., Oliveira, L. C. de, Artigo, C. E., Em, A. A. R., Em, A. A. R., Bachman, L., Chick, K., Curtis, D., Peirce, B. N., Askey, D., Rubin, J., Egnatoff, D. W. J., Uhl Chamot, A., El‐Dinary, P. B., Scott, J.; Marshall, G., Prensky, M., … Santa, U. F. De. (2016b). Budgeting for Local Governments and Communities. In Revista Brasileira de Linguística Aplicada (Vol. 5, Issue 1). https://revistas.ufrj.br/index.php/rce/article/download/1659/1508%0Ahttp://hipatiapress.com/hpjournals/index.php/qre/article/view/1348%5Cnhttp://www.tandfonline.com/doi/abs/10.1080/09500799708666915%5Cnhttps://mckinseyonsociety.com/downloads/reports/Educa
Almeida, I. M. (1995). Desvendando a zona de sombra dos acidentes de trabalho, estudo de acidentes de trabalho graves em Botucatu, SP, no período de 1/1 a 30/6/93 [dissertação de Mestrado]. São Paulo (SP): USP.
Álvarez Rangel, M., & Matos Castro, J. E. (2014). Espiritu empresarial en los programas de Administración de Empresas en las universidades de la ciudad de Cartagena: Herramientas pedagógicas. Universidad de Cartagena.
Alzubaidi, L., Zhang, J., Humaidi, A. J., Al-Dujaili, A., Duan, Y., Al-Shamma, O., Santamaría, J., Fadhel, M. A., Al-Amidie, M., & Farhan, L. (2021). Review of deep learning: concepts, CNN architectures, challenges, applications, future directions. In Journal of Big Data (Vol. 8, Issue 1). Springer International Publishing. https://doi.org/10.1186/s40537-021-00444-8
Antwi-afari, M. F., Li, H., Anwer, S., Kwame, S., Wu, Z., Antwi-afari, P., & Kim, I. (2020). Quantifying workers ’ gait patterns to identify safety hazards in construction using a wearable insole pressure system. Safety Science, 129(December 2019), 104855. https://doi.org/10.1016/j.ssci.2020.104855
Aroquipa, H. (2014). Procesos constructivos de edificaciones y sus impactos ambientales con relación a una producción limpia y sostenible. Tesis Doctoral Universidad nacional del Altiplano, Puno.
Aroquipa, H., & Hurtado, A. (2022a). Seismic resilience assessment of buildings: A simplified methodological approach through conventional seismic risk assessment. International Journal of Disaster Risk Reduction, 77(February), 103047. https://doi.org/10.1016/j.ijdrr.2022.103047
Aroquipa, H., Hurtado, A., Angel, C., Aroquipa, A., Gamarra, A., & Almeida Del Savio, A. (2023). A cost-benefit analysis for the appraisal of social and market prices in the probabilistic seismic risk assessment of building portfolios: A methodology for the evaluation of disaster risk reduction programs. International Journal of Disaster Risk Reduction, 90(October 2022), 103637. https://doi.org/10.1016/j.ijdrr.2023.103637
Aroquipa, H., & Hurtado, A. I. (2022b). Incremental seismic retrofitting for essential facilities using performance objectives: A case study of the 780-PRE school buildings in Peru. Journal of Building Engineering, 62, 105387. https://doi.org/10.1016/j.jobe.2022.105387
Aroquipa Velásquez, H. (2014). Procesos constructivos de edificaciones y sus impactos ambientales con relación a una producción limpia y sostenible.
Arteaga, C., Paz, A., & Park, J. (2020). Injury severity on traffic crashes : A text mining with an interpretable machine-learning approach. Safety Science, 132(September), 104988. https://doi.org/10.1016/j.ssci.2020.104988
Assaf, A. G., & Tsionas, M. (2021). A Bayesian solution to multicollinearity through unobserved common factors. Tourism Management, 84(November 2020), 104277. https://doi.org/10.1016/j.tourman.2020.104277
Assessment and Planning for Resilience | U.S. Green Building Council. (n.d.). Retrieved January 18, 2022, from https://www.usgbc.org/credits/new-construction-core-and-shell-schools-new-construction-retail-new-construction-data-50
Assi, K., Rahman, S. M., Mansoor, U., & Ratrout, N. (2020). Predicting Crash Injury Severity with Machine Learning Algorithm Synergized with Clustering Technique: A Promising Protocol. International Journal of Environmental Research and Public Health, 17(15), 5497. https://doi.org/10.3390/ijerph17155497
Babič, A., & Dolšek, M. (2016). Seismic fragility functions of industrial precast building classes. Engineering Structures, 118, 357–370. https://doi.org/10.1016/j.engstruct.2016.03.069
Bai, Q., & Labi, S. (2009). Uncertainty-Based Tradeoff Analysis Methodology for Integrated Transportation Investment Decision-Making. 92.
Bajpayee, T. S., Rehak, T. R., Mowrey, G. L., & Ingram, D. K. (2004). Blasting injuries in surface mining with emphasis on flyrock and blast area security. Journal of Safety Research, 35(1), 47–57. https://doi.org/10.1016/j.jsr.2003.07.003
Baker, H., Hallowell, M. R., & Tixier, A. J. (2020). AI-based prediction of independent construction safety outcomes from universal attributes. Automation in Construction, 118(August 2019), 103146. https://doi.org/10.1016/j.autcon.2020.103146
Ben-Hur, A., Horn, D., Siegelmann, H. T., & Vapnik, V. (2001). Support vector clustering. Journal of Machine Learning Research, 2(Dec), 125–137.
Bergstra, J., & Bengio, Y. (2012). Random search for hyper-parameter optimization. Journal of Machine Learning Research, 13(2).
Bhattacherjee, A. (1991). Mine safety management: an application of risk analysis, forecasting techniques, and Markov process to injury experience data. The Pennsylvania State University.
Boser, B. E., Guyon, I. M., & Vapnik, V. N. (1992). Training algorithm for optimal margin classifiers. Proceedings of the Fifth Annual ACM Workshop on Computational Learning Theory, 144–152. https://doi.org/10.1145/130385.130401
Breiman, L. (2001). Random forests. Machine Learning, 45, 5–32.
Brink, H., Richards, J., & Fetherolf, M. (2016). Real-world machine learning. Simon and Schuster.
Burnett, R. A., & Si, D. (2017). Prediction of injuries and fatalities in aviation accidents through machine learning. Proceedings of the International Conference on Compute and Data Analysis, 60–68.
Calderon Navarro, M. A. (2015). Optimización de las prácticas de perforación y voladura en el avance y producción de la minería de mediana escala (Unidad Minera Macdesa).
Calderón Solis, A. (2012). Análisis e implementación de un sistema de gestión de riesgos para la prevención de accidentes en la mina El Brocal SAA Unidad Colquijirca-Pasco.
Candia, R. C., Hennies, W. T., Azevedo, R. C., Almeida, I., & Soto, J. (2010). Análisis de accidentes fatales en la industria minera peruana. Boletín Geológico y Minero de España, 1, 57–68.
Cangahuala Sedano, J. A., & Salas Zeballos, V. R. (2022). Sistema de gestión de seguridad y salud ocupacional para la prevención de accidentes laborales en empresas mineras. Llamkasun, 3(1), 112–118. https://doi.org/10.47797/llamkasun.v3i1.90
Carvalho, T. P., Soares, F. A. A. M. N., Vita, R., Francisco, R. da P., Basto, J. P., & Alcalá, S. G. S. (2019). A systematic literature review of machine learning methods applied to predictive maintenance. Computers and Industrial Engineering, 137(September), 106024. https://doi.org/10.1016/j.cie.2019.106024
Castaño Martínez, F. L., Betín, J. M. H., & Sáenz, J. N. G. (2009). Análisis cualitativo del flujo de agua de infiltración para el control del drenaje de una estructura de pavimento flexible. Infraestructura Vial, 1, 6. https://search.ebscohost.com/
Center, N. S. (2013). The case for safety the North Sea Piper alpha disaster. System Failure Case Studies, 7, 1–4.
Chebila, M. (2021a). Predicting the consequences of accidents involving dangerous substances using machine learning. Ecotoxicology and Environmental Safety, 208, 111470.
Chebila, M. (2021b). Predicting the consequences of accidents involving dangerous substances using machine learning. Ecotoxicology and Environmental Safety, 208, 111470. https://doi.org/10.1016/j.ecoenv.2020.111470
Chen, T., & Guestrin, C. (2016). Xgboost: A scalable tree boosting system. Proceedings of the 22nd Acm Sigkdd International Conference on Knowledge Discovery and Data Mining, 785–794.
Cheng, H.-T., Koc, L., Harmsen, J., Shaked, T., Chandra, T., Aradhye, H., Anderson, G., Corrado, G., Chai, W., Ispir, M., Anil, R., Haque, Z., Hong, L., Jain, V., Liu, X., & Shah, H. (2016). Wide & Deep Learning for Recommender Systems. Proceedings of the 1st Workshop on Deep Learning for Recommender Systems, 7–10. https://doi.org/10.1145/2988450.2988454
Chien, K. F., Wu, Z. H., & Huang, S. C. (2014). Identifying and assessing critical risk factors for BIM projects: Empirical study. Automation in Construction, 45, 1–15. https://doi.org/10.1016/j.autcon.2014.04.012
Choi, J., Gu, B., Chin, S., & Lee, J. S. (2020). Machine learning predictive model based on national data for fatal accidents of construction workers. Automation in Construction, 110(September 2019), 102974. https://doi.org/10.1016/j.autcon.2019.102974
Choudhry, R. M., & Iqbal, K. (2013). Identification of Risk Management System in Construction Industry in Pakistan. Journal of Management in Engineering, 29(1), 42–49. https://doi.org/10.1061/(ASCE)ME.1943-5479.0000122
Chung, P. W. H., & Jefferson, M. (1998). The integration of accident databases with computer tools in the chemical industry. Computers & Chemical Engineering, 22, S729–S732. https://doi.org/10.1016/S0098-1354(98)00135-5
Cimellaro, G. P., Reinhorn, A. M., & Bruneau, M. (2010). Seismic resilience of a hospital system. Structure and Infrastructure Engineering, 6(1–2), 127–144. https://doi.org/10.1080/15732470802663847
Conesa, J. A. B. (2014). Implantación de la Responsabilidad Social en la Administración Pública: el caso de las Fuerzas Armadas Españolas. CONSEJO EDITORIAL.
Córdova, M., & Monsalve, C. (2013). Niveles de investigación: Predictiva, proyectiva, interactiva, confirmatoria y evaluativa.
Cortina, A., Conill, J., Domingo, A., & García-Marzá, D. (1996). Ética de la empresa (Issue 174/C82e). Trotta Madrid.
Creswell, J. W., & Creswell, J. D. (2017). Research design: Qualitative, quantitative, and mixed methods approaches. Sage publications.
Cruz, I., & Huerta-Mercado, R. (2015). Occupational Safety and Health in Peru. Annals of Global Health, 81(4), 568. https://doi.org/10.1016/j.aogh.2015.08.027
Del Gaudio, C., Ricci, P., Verderame, G. M., & Manfredi, G. (2015). Development and urban-scale application of a simplified method for seismic fragility assessment of RC buildings. Engineering Structures, 91, 40–57. https://doi.org/10.1016/j.engstruct.2015.01.031
DeVellis, R. F., & Thorpe, C. T. (2021). Scale development: Theory and applications. Sage publications.
Diez-pastor, J. F., Gil, A., Val, D., Veiga, F., & Bustillo, A. (2021). High-accuracy classification of thread quality in tapping processes with ensembles of classifiers for imbalanced learning. Measurement, 168(August 2020), 108328. https://doi.org/10.1016/j.measurement.2020.108328
Dikmen, I., Birgonul, M. T., Tah, J. H. M., & Ozer, A. H. (2012). Web-Based Risk Assessment Tool Using Integrated Duration–Cost Influence Network Model. Journal of Construction Engineering and Management, 138(9), 1023–1034. https://doi.org/10.1061/(ASCE)CO.1943-7862.0000547
do Nascimento Beckert, A., & Barros, V. G. (2022). Waste management, COVID-19 and occupational safety and health: Challenges, insights and evidence. Science of The Total Environment, 831, 154862.
Drummond, C. (2017). Classification. In Encyclopedia of Machine Learning and Data Mining (pp. 205–208). Springer US. https://doi.org/10.1007/978-1-4899-7687-1_111
Elman, J. (1990). Finding structure in time. Cognitive Science, 14(2), 179–211. https://doi.org/10.1016/0364-0213(90)90002-E
Emprey, D. E. (1992). Incoporating management and organizational factors into probabilistic safety assesment. Reliability Engineering and System Safety, 38, 199–208.
Evangelopoulos, N., Zhang, X., & Prybutok, V. R. (2012). Latent Semantic Analysis: five methodological recommendations. European Journal of Information Systems, 21(1), 70–86. https://doi.org/10.1057/ejis.2010.61
Eybpoosh, M., Dikmen, I., & Talat Birgonul, M. (2011). Identification of Risk Paths in International Construction Projects Using Structural Equation Modeling. Journal of Construction Engineering and Management, 137(12), 1164–1175. https://doi.org/10.1061/(ASCE)CO.1943-7862.0000382
Fang, C., & Marle, F. (2012). A simulation-based risk network model for decision support in project risk management. Decision Support Systems, 52(3), 635–644. https://doi.org/10.1016/j.dss.2011.10.021
Feng, S., Keung, J., Yu, X., Xiao, Y., & Zhang, M. (2021). Investigation on the stability of SMOTE-based oversampling techniques in software defect prediction. Information and Software Technology, 139(August 2020), 106662. https://doi.org/10.1016/j.infsof.2021.106662
Firmansyah, H. R., Sarli, P. W., Twinanda, A. P., Santoso, D., & Imran, I. (2024). Building typology classification using convolutional neural networks utilizing multiple ground-level image process for city-scale rapid seismic vulnerability assessment. Engineering Applications of Artificial Intelligence, 131(December 2023), 107824. https://doi.org/10.1016/j.engappai.2023.107824
Flyvbjerg, B. (2013). Quality control and due diligence in project management: Getting decisions right by taking the outside view. International Journal of Project Management, 31(5), 760–774. https://doi.org/10.1016/j.ijproman.2012.10.007
Freund, Y., & Schapire, R. E. (1996). Experiments with a new boosting algorithm. Icml, 96, 148–156.
Friedman, J. H. (2001a). Greedy function approximation: a gradient boosting machine. Annals of Statistics, 1189–1232.
Friedman, J. H. (2001b). Greedy function approximation: A gradient boosting machine. Annals of Statistics, 29(5), 1189–1232. https://doi.org/10.1214/aos/1013203451
García-Acosta Virgina. (2005). El riesgo como construcción social y la construcción social de riesgos. Desacatos, 19, 11–24.
García Navarro, F. (2012). Responsabilidad social corporativa: teoría y práctica. ESIC editorial.
George, E. P., Hunter, W. G., & Hunter, J. S. (2005). Statistics for experimenters: design, innovation, and discovery. Wiley.
Gerassis, S., Saavedra, Á., Taboada, J., Alonso, E., & Bastante, F. G. (2020). Differentiating between fatal and non-fatal mining accidents using artificial intelligence techniques. International Journal of Mining, Reclamation and Environment, 34(10), 687–699. https://doi.org/10.1080/17480930.2019.1700008
Gerón, A. (2023). Aprende machine learning con Scikit-Learn, Keras y TensorFlow. Tercera edición : conceptos, herramientas y técnicas para conseguir sistemas inteligentes. 832.
Giraldo, M., & Badillo, J. (2015). Implicancias técnicas y económicas de los accidentes mortales en la minería peruana. Revista Del Instituto de Investigación (RIIGEO), FIGMMG-UNMSM, 18(35), 97–107.
Giraldo Paredez, E. M. (2016). Identificación de factores para reducir accidentes por desprendimiento de rocas en minería subterránea. Revista Del Instituto de Investigación de La Facultad de Ingeniería Geológica, Minera, Metalurgica y Geográfica, 19(37), 47–55.
Gobbo, J. A., Busso, C. M., Gobbo, S. C. O., & Carreão, H. (2018). Making the links among environmental protection, process safety, and industry 4.0. Process Safety and Environmental Protection, 117, 372–382. https://doi.org/10.1016/j.psep.2018.05.017
Goh, Y. M., Ubeynarayana, C. U., Le, K., Wong, X., & Guo, B. H. W. (2018). Factors in fl uencing unsafe behaviors : A supervised learning approach. 118(June), 77–85. https://doi.org/10.1016/j.aap.2018.06.002
Gómez Rojas, P., Hernández Guerrero, J., & Méndez Campos, M. D. (2014). Factores de riesgo psicosocial y satisfacción laboral en una empresa chilena del área de la minería. Ciencia & Trabajo, 16(49), 9–16.
Goodfellow, I., Bengio, Y., & Courville, A. (2016). Deep learning. MIT press.
Gribaudo, M., Iacono, M., & Kiran, M. (2018). A performance modeling framework for lambda architecture based applications. Future Generation Computer Systems, 86, 1032–1041.
Guzman, J., Arianne, G., Wahid, A., Padrones, J. M., & Jared, J. (2022). Evaluating workplace safety in the oil and gas industry during the COVID-19 pandemic using occupational health and safety Vulnerability Measure and partial least square Structural Equation Modelling. Cleaner Engineering and Technology, 6, 100378. https://doi.org/10.1016/j.clet.2021.100378
Hastie, T., Tibshirani, R., & Friedman, J. (2009). The Elements of Statistical Learning. Springer New York. https://doi.org/10.1007/978-0-387-84858-7
Heravi, G., & Charkhakan, M. H. (2014). Predicting and Tracing Change-Formation Scenarios in Construction Projects Using the DEMATEL Technique. Journal of Management in Engineering, 30(6). https://doi.org/10.1061/(ASCE)ME.1943-5479.0000229
Heravi, G., & Hajihosseini, Z. (2012). Risk Allocation in Public–Private Partnership Infrastructure Projects in Developing Countries: Case Study of the Tehran–Chalus Toll Road. Journal of Infrastructure Systems, 18(3), 210–217. https://doi.org/10.1061/(ASCE)IS.1943-555X.0000090
Hernández-Sampieri, R., Fernández, C., & Batista, P. (2010). Metodología de la Investigación.(5ta. Edición). México: Editorial Mc Graw Hill. Campos, W.(2010). Apuntes de Metodología de La Investigación Científica.
Hernández Sampieri, R., Fernández Collado, C., & Baptista Lucio, P. (2010). Los métodos mixtos. Metodología de La Investigación, 5, 544–599.
Hingorani, R., Tanner, P., Prieto, M., Lara, C., Science, C., & Torroja, E. (2020). Consequence classes and associated models for predicting loss of life in collapse of building structures. Structural Safety, 85(September 2019), 101910. https://doi.org/10.1016/j.strusafe.2019.101910
Hochreiter, S., & Schmidhuber, J. (1997). Long Short-Term Memory. Neural Computation, 9(8), 1735–1780. https://doi.org/10.1162/neco.1997.9.8.1735
Hollnagel, E., Woods, D. D., & Leveson, N. (2006). Resilience engineering: Concepts and precepts. Ashgate Publishing, Ltd.
Hubbard, D. W. (2020). The failure of risk management: Why it’s broken and how to fix it. John Wiley & Sons.
Ilbahar, E., Karaşan, A., Cebi, S., & Kahraman, C. (2018). A novel approach to risk assessment for occupational health and safety using Pythagorean fuzzy AHP & fuzzy inference system. Safety Science, 103(October 2017), 124–136. https://doi.org/10.1016/j.ssci.2017.10.025
Ivakhnenko, A. G. (1971). Polynomial Theory of Complex Systems. IEEE Transactions on Systems, Man and Cybernetics, 1(4), 364–378. https://doi.org/10.1109/TSMC.1971.4308320
James, G., Witten, D., Hastie, T., & Tibshirani, R. (2013). An Introduction to Statistical Learning (Vol. 103). Springer New York. https://doi.org/10.1007/978-1-4614-7138-7
Jang, J. R. (1993). ANFIS : Adap tive-Ne twork-Based Fuzzy Inference System. 23(3).
Janitza, S., Tutz, G., & Boulesteix, A. (2016). Random forest for ordinal responses : Prediction and variable selection. Computational Statistics and Data Analysis, 96, 57–73. https://doi.org/10.1016/j.csda.2015.10.005
Jefferson, M., Chung, P. W. H., & Kletz, T. A. (1997). Learning the lessons from past accidents. Institution of Chemical Engineers Symposium Series, 141, 217–226.
Jelodar, H., Wang, Y., Yuan, C., Feng, X., Jiang, X., Li, Y., & Zhao, L. (2019). Latent Dirichlet allocation (LDA) and topic modeling: models, applications, a survey. Multimedia Tools and Applications, 78(11), 15169–15211. https://doi.org/10.1007/s11042-018-6894-4
Jin, X., & Doloi, H. (2008). Interpreting risk allocation mechanism in public–private partnership projects: an empirical study in a transaction cost economics perspective. Construction Management and Economics, 26(7), 707–721. https://doi.org/10.1080/01446190801998682
Joughin, W. C., Jager, A., Nezomba, E., & Rwodzi, L. (2012). A risk evaluation model for support design in Bushveld Complex underground mines: Part I-Description of the model. Journal of the Southern African Institute of Mining and Metallurgy, 112(2), 83–94.
Jukes, E. (2018). Encyclopedia of Machine Learning and Data Mining (2nd edition). Reference Reviews, 32(7/8), 3–4. https://doi.org/10.1108/RR-05-2018-0084
Kaiser, M. G., El Arbi, F., & Ahlemann, F. (2015). Successful project portfolio management beyond project selection techniques: Understanding the role of structural alignment. International Journal of Project Management, 33(1), 126–139. https://doi.org/10.1016/j.ijproman.2014.03.002
Kalkan, E., & Kunnath, S. K. (2006). Adaptive Modal Combination Procedure for Nonlinear Static Analysis of Building Structures. Journal of Structural Engineering, 132(11), 1721–1731. https://doi.org/10.1061/(asce)0733-9445(2006)132:11(1721)
Kang, K., & Ryu, H. (2019a). Predicting types of occupational accidents at construction sites in Korea using random forest model. Safety Science, 120(January), 226–236. https://doi.org/10.1016/j.ssci.2019.06.034
Kang, K., & Ryu, H. (2019b). Predicting types of occupational accidents at construction sites in Korea using random forest model. Safety Science, 120(January), 226–236. https://doi.org/10.1016/j.ssci.2019.06.034
Ke, G., Meng, Q., Finley, T., Wang, T., Chen, W., Ma, W., Ye, Q., & Liu, T.-Y. (2017). Lightgbm: A highly efficient gradient boosting decision tree. Advances in Neural Information Processing Systems, 30.
Khademi Hamidi, J., Shahriar, K., Rezai, B., Rostami, J., & Bejari, H. (2010). Risk assessment based selection of rock TBM for adverse geological conditions using Fuzzy-AHP. Bulletin of Engineering Geology and the Environment, 69(4), 523–532. https://doi.org/10.1007/s10064-009-0260-8
Khahro, S. H., Memon, Z. A., Gungat, L., Yazid, M. R. M., Rahim, A., Mubaraki, M., & Nur, N. I. (2021). Low-cost pavement management system for developing countries. Sustainability (Switzerland), 13(11). https://doi.org/10.3390/su13115941
Khan, A., Sohail, A., Zahoora, U., & Qureshi, A. S. (2020). A survey of the recent architectures of deep convolutional neural networks. Artificial Intelligence Review, 53(8), 5455–5516. https://doi.org/10.1007/s10462-020-09825-6
Khan, D., & Rawat, A. (2016). Nonlinear Seismic Analysis of Masonry Infill RC Buildings with Eccentric Bracings at Soft Storey Level. Procedia Engineering, 161, 9–17. https://doi.org/10.1016/j.proeng.2016.08.490
Kim, D. Y., Han, S. H., Kim, H., & Park, H. (2009). Structuring the prediction model of project performance for international construction projects: A comparative analysis. Expert Systems with Applications, 36(2 PART 1), 1961–1971. https://doi.org/10.1016/j.eswa.2007.12.048
Kim, H. (2022). Deep Learning. Artificial Intelligence for 6G, 22(4), 247–303. https://doi.org/10.1007/978-3-030-95041-5_6
Kiran, M., Murphy, P., Monga, I., Dugan, J., & Baveja, S. S. (2015a). Lambda architecture for cost-effective batch and speed big data processing. Proceedings - 2015 IEEE International Conference on Big Data, IEEE Big Data 2015, 2785–2792. https://doi.org/10.1109/BigData.2015.7364082
Kiran, M., Murphy, P., Monga, I., Dugan, J., & Baveja, S. S. (2015b). Lambda architecture for cost-effective batch and speed big data processing. 2015 IEEE International Conference on Big Data (Big Data), 2785–2792.
Kletz, T. (2012). The history of process safety. Journal of Loss Prevention in the Process Industries, 25(5), 763–765. https://doi.org/10.1016/j.jlp.2012.03.011
Kletz, T. A. (1993). Lessons from disaster: how organizations have no memory and accidents recur. IChemE.
Kohavi, R. (1995a). A study of cross-validation and bootstrap for accuracy estimation and model selection. Ijcai, 14(2), 1137–1145.
Kohavi, R. (1995b). A Study of Cross-Validation and Bootstrap for Accuracy Estimation and Model Selection. IJCAI International Joint Conference on Artificial Intelligence, 2(March 2001), 1137–1143.
Koldas, K. S. (2001). Rock-related accidents, investigations and inquiries in South African mines. 17th International Mining Congress and Exhibition of Turkey-IMCET.
Kroß, J., Brunnert, A., Prehofer, C., Runkler, T. A., & Krcmar, H. (2015a). Stream processing on demand for lambda architectures. Lecture Notes in Computer Science (Including Subseries Lecture Notes in Artificial Intelligence and Lecture Notes in Bioinformatics), 9272, 243–257. https://doi.org/10.1007/978-3-319-23267-6_16
Kroß, J., Brunnert, A., Prehofer, C., Runkler, T. A., & Krcmar, H. (2015b). Stream processing on demand for lambda architectures. European Workshop on Performance Engineering, 243–257.
Kwon, O. H., Rhee, W., & Yoon, Y. (2015). Application of classification algorithms for analysis of road safety risk factor dependencies. Accident Analysis and Prevention, 75, 1–15. https://doi.org/10.1016/j.aap.2014.11.005
Landucci, G., & Paltrinieri, N. (2016). A methodology for frequency tailorization dedicated to the Oil & Gas sector. Process Safety and Environmental Protection, 104, 123–141. https://doi.org/10.1016/j.psep.2016.08.012
Langstrand, J.-P., Nguyen, H. T., & McDonald, R. (2021). Applying deep learning to solve alarm flooding in digital nuclear power plant control rooms. Advances in Artificial Intelligence, Software and Systems Engineering: Proceedings of the AHFE 2020 Virtual Conferences on Software and Systems Engineering, and Artificial Intelligence and Social Computing, July 16-20, 2020, USA, 521–527.
Lecun, Y., Bottou, L., Bengio, Y., Haffner, P., Lecun, Y., Bottou, L., Bengio, Y., & Haffner, P. (2023). Gradient-based learning applied to document recognition To cite this version : HAL Id : hal-03926082 Gradient-Based Learning Applied to Document Recognition. 86(11), 2278–2324.
Lee, J., Cameron, I., & Hassall, M. (2019). Improving process safety: What roles for digitalization and industry 4.0? Process Safety and Environmental Protection, 132, 325–339. https://doi.org/10.1016/j.psep.2019.10.021
Leeanansaksiri, A., Panyakapo, P., & Ruangrassamee, A. (2018). Seismic capacity of masonry infilled RC frame strengthening with expanded metal ferrocement. Engineering Structures, 159, 110–127. https://doi.org/10.1016/J.ENGSTRUCT.2017.12.034
Li, D., Deng, L., Bhooshan Gupta, B., Wang, H., & Choi, C. (2019). A novel CNN based security guaranteed image watermarking generation scenario for smart city applications. Information Sciences, 479, 432–447. https://doi.org/10.1016/j.ins.2018.02.060
Li, F., Phoon, K. K., Du, X., & Zhang, M. (2013). Improved AHP Method and Its Application in Risk Identification. Journal of Construction Engineering and Management, 139(3), 312–320. https://doi.org/10.1061/(ASCE)CO.1943-7862.0000605
Likun, W., & Zaili, Y. (2018). Bayesian network modelling and analysis of accident severity in waterborne transportation : A case study in China. 180(July), 277–289. https://doi.org/10.1016/j.ress.2018.07.021
Liu, J., Li, Q., & Wang, Y. (2013). Risk analysis in ultra deep scientific drilling project - A fuzzy synthetic evaluation approach. International Journal of Project Management, 31(3), 449–458. https://doi.org/10.1016/j.ijproman.2012.09.015
Liu, R., Liu, Z., Liu, H., & Shi, H. (2021). Automation in Construction An improved alternative queuing method for occupational health and safety risk assessment and its application to construction excavation. Automation in Construction, 126(April 2020), 103672. https://doi.org/10.1016/j.autcon.2021.103672
Lourenço, P., & Cristina, A. (2021). Remote Sensing Applications : Society and Environment Estimating tree aboveground biomass using multispectral satellite-based data in Mediterranean agroforestry system using random forest algorithm. 23(May). https://doi.org/10.1016/j.rsase.2021.100560
Lowe, D. S. B. and D. (1988). Multivariable Functional Interpolation and Adaptive Networks. Complex Systems, 2, 321–355.
Lu, J. L. (2022). Mining safety and health in the Philippines: Occupational and environmental impacts. Safety and Health at Work, 13, S142.
Lu, J., Su, W., Jiang, M., & Ji, Y. (2022). Severity prediction and risk assessment for non-traditional safety events in sea lanes based on a random forest approach. Ocean and Coastal Management, 225(February), 106202. https://doi.org/10.1016/j.ocecoaman.2022.106202
Luo, X., Cruz, A. M., & Tzioutzios, D. (2020). Extracting Natech Reports from Large Databases: Development of a Semi-Intelligent Natech Identification Framework. International Journal of Disaster Risk Science, 11(6), 735–750. https://doi.org/10.1007/s13753-020-00314-6
Luo, X., Li, X., Goh, Y. M., Song, X., & Liu, Q. (2023). Application of machine learning technology for occupational accident severity prediction in the case of construction collapse accidents. Safety Science, 163(March), 106138. https://doi.org/10.1016/j.ssci.2023.106138
Lyu, H.-M., Sun, W.-J., Shen, S.-L., & Zhou, A.-N. (2020). Risk Assessment Using a New Consulting Process in Fuzzy AHP. Journal of Construction Engineering and Management, 146(3). https://doi.org/10.1061/(ASCE)CO.1943-7862.0001757
Mannan, M. S., & Waldram, S. P. (2014). Learning lessons from incidents: A paradigm shift is overdue. Process Safety and Environmental Protection, 92(6), 760–765. https://doi.org/10.1016/j.psep.2014.02.001
Manning, C. D. (2008). Introduction to information retrieval. Syngress Publishing,.
Marcelino-Sádaba, S., Pérez-Ezcurdia, A., Echeverría Lazcano, A. M., & Villanueva, P. (2014). Project risk management methodology for small firms. International Journal of Project Management, 32(2), 327–340. https://doi.org/10.1016/j.ijproman.2013.05.009
Martínez, A. V., Unanue, R. M., & Yuste, Á. R. (2015). Arquitectura lambda aplicada a clustering de documentos en contextos Big Data.
Mazaira, A., & Konicek, P. (2015). Intense rockburst impacts in deep underground construction and their prevention. Canadian Geotechnical Journal, 52(10), 1426–1439.
Medina, A. B. C. (2014). Investigación holística y Desarrollo Instruccional en la comprensión del discurso escrito en estudiantes de educación media de la UEN José Félix Blanco. Caracas. Revista de Investigación, 38(81), 69–88.
Mejía, C. R., Merino, P. A., Mescua, L., & Gomero, R. (2015). Notificación de accidentes mortales en el sector de minería Peruana, 2000-2014. Accidentes mortales en minería peruana. Archivos de Medicina, 11(4), 11.
Mikolov, T., Sutskever, I., Chen, K., Corrado, G. S., & Dean, J. (2013). Distributed representations of words and phrases and their compositionality. Advances in Neural Information Processing Systems, 26.
Mileti, D. (1999). Disasters by Design. In Disasters by Design (Issue April). https://doi.org/10.17226/5782
Moreno Chacón, N. (2011). Modelo de un programa de seguridad e higiene para la minería subterránea del carbón en Colombia.
Moretti, L., Cantisani, G., & Di Mascio, P. (2016). Management of road tunnels: Construction, maintenance and lighting costs. Tunnelling and Underground Space Technology, 51, 84–89. https://doi.org/10.1016/j.tust.2015.10.027
Murphy, K. P. (2012). Machine learning: a probabilistic perspective. MIT press.
Musell, R. M., & Yeung, R. (2019). Understanding Government Budgets. Routledge. https://doi.org/10.4324/9781315474854
Mutlu, N. G., & Altuntas, S. (2019). International Journal of Industrial Ergonomics Risk analysis for occupational safety and health in the textile industry : Integration of FMEA , FTA , and BIFPET methods. International Journal of Industrial Ergonomics, 72(June), 222–240. https://doi.org/10.1016/j.ergon.2019.05.013
Noa Monjes, R. R. (2006). Indicaciones metodológicas para la elección del método de arranque de las rocas durante el laboreo de excavaciones subterráneas horizontales de pequeña y mediana sección en Cuba oriental. Minería y Geología, 22(4), 50.
Paltrinieri, N., Dechy, N., Salzano, E., Wardman, M., & Cozzani, V. (2013). Towards a new approach for the identification of atypical accident scenarios. Journal of Risk Research, 16(3–4), 337–354. https://doi.org/10.1080/13669877.2012.729518
Paltrinieri, N., Patriarca, R., Stefana, E., Brocal, F., & Reniers, G. (2020). Meta-learning for safety management. Chemical Engineering Transactions, 82, 169–174.
Pan, T., Zhao, J., Wu, W., & Yang, J. (2020). Learning imbalanced datasets based on SMOTE and Gaussian distribution. Information Sciences, 512, 1214–1233. https://doi.org/10.1016/j.ins.2019.10.048
Paolanti, M., Romeo, L., Felicetti, A., Mancini, A., Frontoni, E., & Loncarski, J. (2018). Machine Learning approach for Predictive Maintenance in Industry 4.0. 2018 14th IEEE/ASME International Conference on Mechatronic and Embedded Systems and Applications (MESA), 1–6. https://doi.org/10.1109/MESA.2018.8449150
Parinaz Jafari, Malak Al Hattab, E. M., & Simaan AbouRizk. (2021). Automated Extraction and Time-Cost Prediction of Contractual Reporting Requirements in Construction Using Natural Language Processing and Simulation. MDPI. https://doi.org/doi.org/10.3390/app11136188
Pasman, H. J. (2009). Learning from the past and knowledge management: Are we making progress? Journal of Loss Prevention in the Process Industries, 22(6), 672–679. https://doi.org/10.1016/j.jlp.2008.07.010
Pasman, H. J., Duxbury, H. A., & Bjordal, E. N. (1992). Major hazards in the process industries: Achievements and challenges in loss prevention. Journal of Hazardous Materials, 30(1), 1–38. https://doi.org/10.1016/0304-3894(92)87072-N
Pasman, H. J., & Fabiano, B. (2021). The Delft 1974 and 2019 European Loss Prevention Symposia: Highlights and an impression of process safety evolutionary changes from the 1st to the 16th LPS. Process Safety and Environmental Protection, 147, 80–91. https://doi.org/10.1016/j.psep.2020.09.024
Paul, P. S., & Maiti, J. (2007). The role of behavioral factors on safety management in underground mines. Safety Science, 45(4), 449–471.
Pérez, J. L. (2007). Sistema de gestión en seguridad y salud ocupacional aplicado a empresas contratistas en el sector económico minero metalúrgico.
Phark, C., Kim, W., Yoon, Y. S., Shin, G., & Jung, S. (2018). Prediction of issuance of emergency evacuation orders for chemical accidents using machine learning algorithm. Journal of Loss Prevention in the Process Industries, 56(March), 162–169. https://doi.org/10.1016/j.jlp.2018.08.021
Poh, C. Q. X., Udhyami, C., & Miang, Y. (2018). Automation in Construction Safety leading indicators for construction sites : A machine learning approach. Automation in Construction, 93(March), 375–386. https://doi.org/10.1016/j.autcon.2018.03.022
Prokhorenkova, L., Gusev, G., Vorobev, A., Dorogush, A. V., & Gulin, A. (2018). CatBoost: unbiased boosting with categorical features. Advances in Neural Information Processing Systems, 31.
Provost, F., & Fawcett, T. (2013). Data Science for Business: What you need to know about data mining and data-analytic thinking. “ O’Reilly Media, Inc.”
Qazi, A., Quigley, J., Dickson, A., & Kirytopoulos, K. (2016). Project Complexity and Risk Management (ProCRiM): Towards modelling project complexity driven risk paths in construction projects. International Journal of Project Management, 34(7), 1183–1198. https://doi.org/10.1016/j.ijproman.2016.05.008
Qian, W., Huang, J., Wang, Y., & Shu, W. (2020). Knowledge-Based Systems Mutual information-based label distribution feature selection for. Knowledge-Based Systems, 195, 105684. https://doi.org/10.1016/j.knosys.2020.105684
Rajasekaran, S., Gayathri, S., & Lee, T. L. (2008). Support vector regression methodology for storm surge predictions. Ocean Engineering, 35(16), 1578–1587. https://doi.org/10.1016/j.oceaneng.2008.08.004
Reason, J. (1990). James Reason : Human Error Person approach Evaluating the person approach. 1–7.
Retrieval, I. (2009). Introduction to information retrieval. In Choice Reviews Online (Vol. 46, Issue 05). https://doi.org/10.5860/choice.46-2715
Rezakhani, P., Jang, W.-S., Lee, S., & Lee, D.-E. (2014). Project risk assessment model combining the fuzzy weighted average principle with a similarity measure. KSCE Journal of Civil Engineering, 18(2), 521–530. https://doi.org/10.1007/s12205-014-0053-x
Rinc, I. B. (2011). Investigación científica e investigación tecnológica como componentes para la innovación: consideraciones técnicas y metodológicas. 220–255.
Robert F. DeVellis. (2019). Frank D. Brown Hall Room 3208. To Achieve Excellence by Guiding Individuals as They Become Professionals”, 1–7.
Rosenblatt, F. (2021). The Perceptron: A Probabilistic Model for Information Storage and Organization (1958). Ideas That Created the Future, 65(1958), 183–190. https://doi.org/10.7551/mitpress/12274.003.0020
Rumelhart, D. E., Hinton, G. E., & Williams, R. J. (1986). Learning representations by back-propagating errors. Nature, 323(6088), 533–536. https://doi.org/10.1038/323533a0
Sarkar, S., & Maiti, J. (2020a). Machine learning in occupational accident analysis : A review using science mapping approach with citation network analysis. Safety Science, 131(September 2019), 104900. https://doi.org/10.1016/j.ssci.2020.104900
Sarkar, S., & Maiti, J. (2020b). Machine learning in occupational accident analysis: A review using science mapping approach with citation network analysis. Safety Science, 131(June), 104900. https://doi.org/10.1016/j.ssci.2020.104900
Sarkar, S., Pramanik, A., Maiti, J., & Reniers, G. (2020). Predicting and analyzing injury severity: A machine learning-based approach using class-imbalanced proactive and reactive data. Safety Science, 125(February), 104616. https://doi.org/10.1016/j.ssci.2020.104616
Sasaki, Y. (2007). The truth of the f-measure. 2007. URL: Https://Www. Cs. Odu. Edu/Mukka/Cs795sum09dm/Lecturenotes/Day3/F-Measure-YS-26Oct07. Pdf [Accessed 2021-05-26], 49.
Schmidhuber, J. (2015). Deep Learning in neural networks: An overview. Neural Networks, 61, 85–117. https://doi.org/10.1016/j.neunet.2014.09.003
Schwalbe, K. (2016). Information technology project management. Cengage Learning.
Simard, M., & Marchand, A. (1995). A multilevel analysis of organisational factors related to the taking of safety initiatives by work groups. Safety Science, 21(2), 113–129.
Song, L., & Yang, L. (2021). Governance innovation of occupational safety and health in China under the background of industry 4.0. Industrial Safety and Environmental Protection, 47(09), 79–82.
Sony, S., Dunphy, K., Sadhu, A., & Capretz, M. (2021). A systematic review of convolutional neural network-based structural condition assessment techniques. Engineering Structures, 226(January 2020), 111347. https://doi.org/10.1016/j.engstruct.2020.111347
Soto, I. B. R. (2011). Investigación científica e investigación tecnológica como componentes para la innovación: consideraciones técnicas y metodológicas. Contribuciones a Las Ciencias Sociales, 8.
Stemn, E., & Krampah, F. (2022). Injury severity and influence factors in surface mines : A correspondence analysis. Safety Science, 145(September 2021), 105495. https://doi.org/10.1016/j.ssci.2021.105495
Stone, P. (2017). Reinforcement Learning. In Encyclopedia of Machine Learning and Data Mining (pp. 1088–1090). Springer US. https://doi.org/10.1007/978-1-4899-7687-1_720
Tamascelli, N., Scarponi, G., Paltrinieri, N., & Cozzani, V. (2021). A data-driven approach to improve control room operators’ response.
Tamascelli, N., Solini, R., Paltrinieri, N., & Cozzani, V. (2022). Learning from major accidents : A machine learning approach. Computers and Chemical Engineering, 162(July 2021), 107786. https://doi.org/10.1016/j.compchemeng.2022.107786
Tavakolan, M., & Etemadinia, H. (2017). Fuzzy Weighted Interpretive Structural Modeling: Improved Method for Identification of Risk Interactions in Construction Projects. Journal of Construction Engineering and Management, 143(11). https://doi.org/10.1061/(ASCE)CO.1943-7862.0001395
Terán Pareja, I. S. (2012). Propuesta de implementación de un sistema de gestión de seguridad y salud ocupacional bajo la norma OHSAS 18001 en una empresa de capacitación técnica para la industria.
Tharwat, A. (2019). Parameter investigation of support vector machine classifier with kernel functions. Knowledge and Information Systems, 61, 1269–1302.
Thorvaldsen, T., Kongsvik, T., Marie, I., Størkersen, K., Salomonsen, C., Sandsund, M., & Bjelland, H. V. (2020). Occupational health , safety and work environments in Norwegian fi sh farming - employee perspective. Aquaculture, 524(December 2019), 735238. https://doi.org/10.1016/j.aquaculture.2020.735238
Tixier, A. J., Hallowell, M. R., Rajagopalan, B., & Bowman, D. (2020). Automation in Construction Automated content analysis for construction safety : A natural language processing system to extract precursors and outcomes from unstructured injury reports. Automation in Construction, 62(2016), 45–56. https://doi.org/10.1016/j.autcon.2015.11.001
Tixier, A. J. P., Hallowell, M. R., Rajagopalan, B., & Bowman, D. (2016). Automated content analysis for construction safety: A natural language processing system to extract precursors and outcomes from unstructured injury reports. Automation in Construction, 62(2016), 45–56. https://doi.org/10.1016/j.autcon.2015.11.001
Unidas, N. (2003). Congestión de tránsito: el problema y cómo enfrentarlo. CEPAL: División de Medio Ambiente y Asentamientos Humanos. https://repositorio.cepal.org/handle/11362/27813
Vaiman, Bell, Chen, Chowdhury, Dobson, Hines, Papic, Miller, & Zhang. (2012). Risk Assessment of Cascading Outages: Methodologies and Challenges. IEEE Transactions on Power Systems, 27(2), 631–641. https://doi.org/10.1109/TPWRS.2011.2177868
Vapnik, V. (2013). The nature of statistical learning theory. Springer science & business media.
Viteri, N. C. (2012). LA INVESTIGACIÓN MIXTA, ESTRATEGIA ANDRAGÓGICA FUNDAMENTAL PARA FORTALECER LAS CAPACIDADES INTELECTUALES SUPERIORES. REVISTA CIENTÍFICA VOL 2, NÚMERO 2, AGOSTO DE 2012.
Wahab, L., & Jiang, H. (2019). A comparative study on machine learning based algorithms for prediction of motorcycle crash severity. PLoS One, 14(4), e0214966.
Wang, J., & Yuan, H. (2017). System Dynamics Approach for Investigating the Risk Effects on Schedule Delay in Infrastructure Projects. Journal of Management in Engineering, 33(1). https://doi.org/10.1061/(ASCE)ME.1943-5479.0000472
Wang, L., & Zhang, X. (2018). Bayesian Analytics for Estimating Risk Probability in PPP Waste-to-Energy Projects. Journal of Management in Engineering, 34(6). https://doi.org/10.1061/(ASCE)ME.1943-5479.0000658
Wang, W., & Yang, Y. (2019). Development of convolutional neural network and its application in image classification: a survey. Optical Engineering, 58(04), 1. https://doi.org/10.1117/1.oe.58.4.040901
Welcome to Python.org. (2024). https://www.python.org/
Wright, C. (1986). Routine deaths: Fatal accidents in the oil industry. The Sociological Review, 34(2), 265–289.
Wu, N. J. (2021). Predicting the compressive strength of concrete using an rbf-ann model. Applied Sciences (Switzerland), 11(14). https://doi.org/10.3390/app11146382
Xu, N., Ma, L., Liu, Q., Wang, L., & Deng, Y. (2021). An improved text mining approach to extract safety risk factors from construction accident reports. 138(February). https://doi.org/10.1016/j.ssci.2021.105216
Xu, Q., Chong, H., & Liao, P. (2019). Exploring eye-tracking searching strategies for construction hazard recognition in a laboratory scene. Safety Science, 120(August), 824–832. https://doi.org/10.1016/j.ssci.2019.08.012
Xu, Z., & Saleh, J. H. (2021). Machine learning for reliability engineering and safety applications: Review of current status and future opportunities. Reliability Engineering and System Safety, 211(December 2020), 107530. https://doi.org/10.1016/j.ress.2021.107530
Yan, X., Xiong, X., Cheng, X., Huang, Y., & Zhu, H. (2021). HMM-BiMM : Hidden Markov Model-based word segmentation via improved Bi-directional Maximal Matching algorithm ✩. Computers and Electrical Engineering, 94(July), 107354. https://doi.org/10.1016/j.compeleceng.2021.107354
Yang, K., & Ahn, C. R. (2019). Advanced Engineering Informatics Inferring workplace safety hazards from the spatial patterns of workers ’ wearable data. Advanced Engineering Informatics, 41(January), 100924. https://doi.org/10.1016/j.aei.2019.100924
Yedla, A., Kakhki, F. D., & Jannesari, A. (2020). Predictive Modeling for Occupational Safety Outcomes and Days Away from Work Analysis in Mining Operations. International Journal of Environmental Research and Public Health, 17(19), 7054. https://doi.org/10.3390/ijerph17197054
Yim, R., Castaneda, J., Doolen, T., Tumer, I., & Malak, R. (2015). A study of the impact of project classification on project risk indicators. International Journal of Project Management, 33(4), 863–876. https://doi.org/10.1016/j.ijproman.2014.10.005
Yodo, N., & Wang, P. (2016). Resilience modeling and quantification for engineered systems using Bayesian networks. Journal of Mechanical Design, 138(3), 31404.
yoshua bengio. (2019). Deep learning 简介 一 、 什么是 Deep Learning ?. Nature, 29(7553), 1–73.
Yusri, A. Z. dan D. (2020). 済無No Title No Title No Title. Jurnal Ilmu Pendidikan, 7(2), 809–820.
Zhang, F. (2022). A hybrid structured deep neural network with Word2Vec for construction accident causes classification. International Journal of Construction Management, 22(6), 1120–1140.
Zhang, J., & El-Gohary, N. M. (2017). Integrating semantic NLP and logic reasoning into a unified system for fully-automated code checking. Automation in Construction, 73, 45–57. https://doi.org/10.1016/j.autcon.2016.08.027
Zhang, J., Li, Z., Pu, Z., & Xu, C. (2018). Comparing Prediction Performance for Crash Injury Severity Among Various Machine Learning and Statistical Methods. IEEE Access, 6, 60079–60087. https://doi.org/10.1109/ACCESS.2018.2874979
Zhao, B., Liu, Y., Goh, S. H., & Lee, F. H. (2016). Parallel finite element analysis of seismic soil structure interaction using a PC cluster. Computers and Geotechnics, 80, 167–177. https://doi.org/10.1016/j.compgeo.2016.07.006
Zhao, X., Hwang, B. G., & Gao, Y. (2016). A fuzzy synthetic evaluation approach for risk assessment: A case of Singapore’s green projects. Journal of Cleaner Production, 115(January 2005), 203–213. https://doi.org/10.1016/j.jclepro.2015.11.042
Zheng, X., Easa, S. M., Ji, T., & Jiang, Z. (2020). Incorporating uncertainty into life-cycle sustainability assessment of pavement alternatives. Journal of Cleaner Production, 264(April). https://doi.org/10.1016/j.jclepro.2020.121466
Zope, K., Singh, K., Nistala, S. H., Basak, A., Rathore, P., & Runkana, V. (2019). Anomaly detection and diagnosis in manufacturing systems: A comparative study of statistical, machine learning and deep learning techniques. Proceedings of the Annual Conference of the Prognostics and Health Management Society, PHM, 11(1), 1–10. https://doi.org/10.36001/phmconf.2019.v11i1.815
Zorzenon, R., Lizarelli, F. L., & Moura, D. B. A. D. A. (2022). What is the potential impact of industry 4 . 0 on health and safety at work ? Safety Science, 153(February), 105802. https://doi.org/10.1016/j.ssci.2022.105802
Zou, Y., Kiviniemi, A., & Jones, S. W. (2016). Developing a tailored RBS linking to BIM for risk management of bridge projects. Engineering, Construction and Architectural Management, 23(6), 727–750. https://doi.org/10.1108/ECAM-01-2016-0009
Zou, Y., Kiviniemi, A., & Jones, S. W. (2017). Retrieving similar cases for construction project risk management using Natural Language Processing techniques. Automation in Construction, 80(February), 66–76. https://doi.org/10.1016/j.autcon.2017.04.003
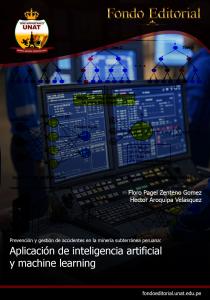
Publicado
Colección
Categorías
Licencia

Esta obra está bajo una licencia internacional Creative Commons Atribución-NoComercial-SinDerivadas 4.0.